可解释机器学习最新综述:应用于实时场景中的机遇与挑战
大数据文摘授权转载自将门创投
作者:Guanchu Wang
论文链接:
什么是可解释机器学习?
因此,本文所讨论的解释算法都是样本层面的解释,不涉及模型层面的解释。

实时系统需要怎样的模型解释算法?
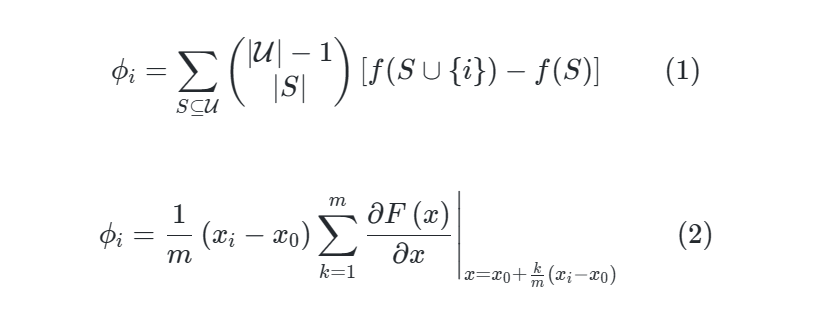

图 2 模型解释加速算法的分类。
非批量解释的加速方法
批量解释的方法
总的来说,批量解释方法假设模型解释的结果服从某种分布,这种分布可以通过一个全局解释器来学习。学习过程在训练集上进行,训练好的解释器可以在实际场景中批量生成解释。批量解释方法是模型解释加速的质的飞跃。
批量模型解释的技术路线有:基于预测模型、基于生成模型和基于强化学习等。下面详细叙述每一条技术路线的代表工作。
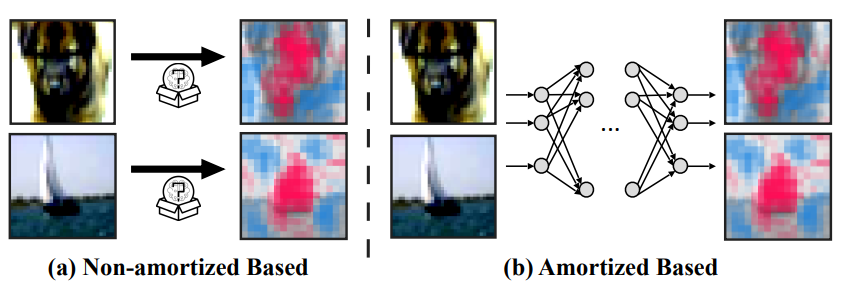
现有工作的局限性及未来研究的难点
对原模型采样更多的预测值可以提高解释的精度,但是会降低解释生成的速度。此外,批量解释方法需要在训练集上学习全局解释器。训练过程的时间及空间消耗和训练精度之间也存在类似的平衡关系。已有工作为可解释机器学习提供了基准,未来解释算法需要能达到性能和速度二者更优的平衡。
结束语
http://128.84.21.203/abs/2302.03225
[1] Chuang Y N, Wang G, Yang F, et al. “Efficient XAI Techniques: A Taxonomic Survey.” arXiv preprint arXiv:2302.03225, 2023.
[2] J. Chen, L. Song, M. J. Wainwright, and M. I. Jordan, “L-shapley and c-shapley: Efficient model interpretation for structured data,” arXiv preprint arXiv:1808.02610, 2018.
[3] Rubinstein R Y, Kroese D P. “Simulation and the Monte Carlo method.” John Wiley & Sons, 2016.
[4] R. Mitchell, J. Cooper, E. Frank, and G. Holmes, “Sampling permutations for shapley value estimation,” 2022.
[5] S. M. Lundberg, G. Erion, H. Chen, A. DeGrave, J. M. Prutkin, B. Nair, R. Katz, J. Himmelfarb, N. Bansal, and S.-I. Lee, “From local explanations to global understanding with explainable ai for trees,” Nature machine intelligence, vol. 2, no. 1, pp. 56–67, 2020.
[6] Hesse R, Schaub-Meyer S, Roth S. “Fast axiomatic attribution for neural networks.” Advances in Neural Information Processing Systems, 2021.
[7] Chen J, Song L, Wainwright M, et al. “Learning to explain: An information-theoretic perspective on model interpretation”, International Conference on Machine Learning. PMLR, 2018.
[8] N. Jethani, M. Sudarshan, I. C. Covert, S.-I. Lee, and R. Ranganath, “Fastshap: Real-time shapley value estimation,” in International Conference on Learning Representations, 2021
[9] Yu-Neng Chuang, Guanchu Wang, Fan Yang, Quan Zhou, Pushkar Tripathi, Xuanting Cai, Xia Hu, “CoRTX: Contrastive Framework for Real-time Explanation,” in International Conference on Learning Representations, 2022.
[10] Yang F, Alva S S, Chen J, et al. “Model-based counterfactual synthesizer for interpretation,” Proceedings of the 27th ACM SIGKDD conference on knowledge discovery & data mining. 2021.
[11] Rodriguez, Pau, et al. “Beyond trivial counterfactual explanations with diverse valuable explanations.” Proceedings of the IEEE/CVF International Conference on Computer Vision. 2021.
[12] Chen, Ziheng, et al. “ReLAX: Reinforcement Learning Agent Explainer for Arbitrary Predictive Models.” Proceedings of the 31st ACM International Conference on Information & Knowledge Management. 2022.
[13] Verma, Sahil, Keegan Hines, and John P. Dickerson. “Amortized generation of sequential algorithmic recourses for black-box models.” Proceedings of the AAAI Conference on Artificial Intelligence. Vol. 36. No. 8. 2022.
[14] Lundberg, Scott M., and Su-In Lee. “A unified approach to interpreting model predictions.” Advances in neural information processing systems, 2017.
[15] Ribeiro, Marco Tulio, Sameer Singh, and Carlos Guestrin. “Why should i trust you? Explaining the predictions of any classifier.” Proceedings of the 22nd ACM SIGKDD international conference on knowledge discovery and data mining. 2016.
[16] Sundararajan, Mukund, Ankur Taly, and Qiqi Yan. “Axiomatic attribution for deep networks.” International conference on machine learning. PMLR, 2017.

关注公众号:拾黑(shiheibook)了解更多
[广告]赞助链接:
四季很好,只要有你,文娱排行榜:https://www.yaopaiming.com/
让资讯触达的更精准有趣:https://www.0xu.cn/
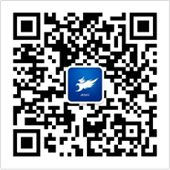
随时掌握互联网精彩
- 1 习近平对西藏6.8级地震作出指示 7981932
- 2 刘强东给乡亲发钱 运钞车到场 7910665
- 3 张雪峰:女儿去哪过亿存款就存哪 7802561
- 4 流感高发 家庭消毒别再这样做了 7797801
- 5 景区回应贪睡出名的鱼真死了 7654685
- 6 怎么舒淇还长这样啊 7544247
- 7 #为何日喀则地震伤亡会这么大# 7443137
- 8 演员王星在飞机上自述失联过程 7381810
- 9 《还珠格格》剧情太超前了 7289773
- 10 手机价格不超6000元可获补贴 7128656