跨越重重“障碍”,我从 PyTorch 转换为了 TensorFlow Lite

本文最初发表在 Towards Data Science 博客,经原作者 Ran Rubin 授权,InfoQ 中文站翻译并分享。
我最近不得不将深度学习模型(MobileNetV2 的变体)从 PyTorch 转换为 TensorFlow Lite。这是一个漫长而复杂的旅程。需要跨越很多障碍才能成功。我发现自己从 StackOverflow 帖子和 GitHub 的问题中搜集了一些信息。我的目标是分享我的经验,以帮助其他像我一样“迷失”的人。
免责声明:本文并非关于如何正确进行转换的指南。我只想分享我的经验,但我也有可能做错(尤其是因为我没有 TensorFlow 的经验)。
将深度学习模型(MobileNetV2 变体)从 PyTorch 转换为 TensorFlow Lite,转换过程应该是这样的:
PyTorch → ONNX → TensorFlow → TFLite
为了测试转换后的模型,我生成了一组大约 1000 个输入张量,并为每个模型计算了 PyTorch 模型的输出。这个集合后来被用来测试每个转换后的模型,方法是通过一个平均误差度量,在整个集合中将它们的输出与原始输出进行比较。在相同的输入下,平均误差反映了在相同的输入下,转换后的模型输出与原始 PyTorch 模型输出相比有多大的不同。
我决定将平均误差小于 1e-6 的模型视为成功转换的模型。
可能还需要注意的是,我在张量中添加了批维度,尽管它为 1。我没有理由这么做,除了来自我以前将 PyTorch 转换为 DLC 模型 的经验的直觉。
这绝对是最简单的部分。这主要归功于 PyTorch 的优秀文档,例如 TORCH.ONNX 的文档 和《(可选)将模型从 PyTorch 导出到 ONNX 并使用 ONNX 运行时运行》((Optional) Exporting a model from pytorch to onnx and running it using onnx runtime)。
要求:
ONNX == 1.7.0 PyTorch == 1.5.1
import onnx
import torch
example_input = get_example_input() # exmample for the forward pass input
pytorch_model = get_pytorch_model()
ONNX_PATH="./my_model.onnx"
torch.onnx.export(
model=pytorch_model,
args=example_input,
f=ONNX_PATH, # where should it be saved
verbose=False,
export_params=True,
do_constant_folding=False, # fold constant values for optimization
# do_constant_folding=True, # fold constant values for optimization
input_names=['input'],
output_names=['output']
)
onnx_model = onnx.load(ONNX_PATH)
onnx.checker.check_model(onnx_model)
Python 到 ONNX 的转换
新创建的 ONNX 模型在我的示例输入上进行了测试,得到的平均误差为 1.39e-06。
请注意,你必须将torch.tensor
示例转换为它们的等效np.array
,才能通过 ONNX 模型运行它。
现在,我有了 ONNX 模型,为了转换成 TensorFlow,我使用了 ONNX-TensorFlow(v1.6.0)库。我并没有使用 TensorFlow 的经验,所以我知道这是事情变得有挑战性的地方。
要求:
TensorFlow == 2.2.0(这是 onnx-tensorflow 的先决条件。不过,它也适用于 tf-nightly 版本 2.4.0-dev20200923
)。tensorflow-addons == 0.11.2 onnx-tensorflow==1.6.0
我也不知道为什么,但这种转换只能用在我的 GPU 机器。
from onnx_tf.backend import prepare
import onnx
TF_PATH = "./my_tf_model.pb" # where the representation of tensorflow model will be stored
ONNX_PATH = "./my_model.onnx" # path to my existing ONNX model
onnx_model = onnx.load(ONNX_PATH) # load onnx model
# prepare function converts an ONNX model to an internel representation
# of the computational graph called TensorflowRep and returns
# the converted representation.
tf_rep = prepare(onnx_model) # creating TensorflowRep object
# export_graph function obtains the graph proto corresponding to the ONNX
# model associated with the backend representation and serializes
# to a protobuf file.
tf_rep.export_graph(TF_PATH)
ONNX 到 TensorFlow 的转换我在创建的 对象运行了测试(这里是使用它进行推理的示例)。运行 超级慢(大约有 1 小时,而不是几秒钟!),所以这让我很担心。然而,最终测试的平均误差为 6.29e-07,所以我决定继续。
此时最大的问题是——它导出了什么?这个.pb
文件又是什么?
我在网上搜索一番后,才意识到这是tf.Graph
的一个实例。现在 剩下要做的就是 把它转换成 TensorFlow Lite。
这就是事情对我来说非常棘手的地方。据我所知,TensorFlow 提供了 3 种方法来将 TF 转换为 TFLite:SavedModel、Keras 和具体函数。可是我不太熟悉这些选项,但我已经知道 onnx-tensorflow 工具导出的内容是一个冻结的图,所以,这三个选项都帮不了我。
我在网上搜索了很久之后,这个家伙 基本上拯救了我。原来,TensorFlowv1
是支持从冻结图进行转换的!我决定在剩下的代码中使用v1
API。
在运行转换函数时,出现了一个奇怪的问 p 题,它与protobuf
库有关。遵循 这个用户 的建议,我得以能够继续前进。
TF_PATH = "./my_tf_model.pb" # where the forzen graph is stored
TFLITE_PATH = "./my_model.tflite"
# protopuf needs your virtual environment to be explictly exported in the path
os.environ["PATH"] = "/opt/miniconda3/envs/convert/bin:/opt/miniconda3/bin:/usr/local/sbin:...."
# make a converter object from the saved tensorflow file
converter = tf.compat.v1.lite.TFLiteConverter.from_frozen_graph(TF_PATH, # TensorFlow freezegraph .pb model file
input_arrays=['input'], # name of input arrays as defined in torch.onnx.export function before.
output_arrays=['output'] # name of output arrays defined in torch.onnx.export function before.
)
# tell converter which type of optimization techniques to use
# to view the best option for optimization read documentation of tflite about optimization
# go to this link https://www.tensorflow.org/lite/guide/get_started#4_optimize_your_model_optional
# converter.optimizations = [tf.compat.v1.lite.Optimize.DEFAULT]
converter.experimental_new_converter = True
# I had to explicitly state the ops
converter.target_spec.supported_ops = [tf.compat.v1.lite.OpsSet.TFLITE_BUILTINS,
tf.compat.v1.lite.OpsSet.SELECT_TF_OPS]
tf_lite_model = converter.convert()
# Save the model.
with open(TFLITE_PATH, 'wb') as f:
f.write(tf_lite_model)
TF 冻结图到 TFLite你可能会认为,在经历了所有这些麻烦之后,在新创建的tflite
模型上运行 推理 可以平静地进行。但是,我的麻烦并没有就此结束,更多的问题出现了。
其中之一与名为“ops”的东西有关(一个带有“Ops that can be supported by the Flex.
”的错误消息)。经过一番搜索,我才意识到,我的模型架构需要在转换之前 显式地启用一些操作符(见上文)。
然后,我发现我的网络使用的许多操作仍在开发中,因此正在运行的 TensorFlow 版本 2.2.0 无法识别它们。通过安装 TensorFlow 的 nightly 版本(特别是nightly==2.4.0.dev20299923
),才解决了这一问题。
我遇到的另一个错误是“The Conv2D op currently only supports the NHWC tensor format on the CPU. The op was given the format: NCHW
”,在 这位用户的评论 的帮助下,这个问题得到了解决。
最后,下面是用于测试的推理代码:
import os
import tensorflow as tf
import numpy as np
TFLITE_PATH = "./my_model.tflite"
example_input = get_numpy_example()
print(f"Using tensorflow {tf.__version__}") # make sure it's the nightly build
os.environ["CUDA_VISIBLE_DEVICES"] = "-1"
interpreter = tf.compat.v1.lite.Interpreter(model_path=TFLITE_PATH)
interpreter.allocate_tensors()
input_details = interpreter.get_input_details()
output_details = interpreter.get_output_details()
interpreter.set_tensor(input_details[0]('index'), example_input)
interpreter.invoke()
print(interpreter.get_tensor(output_details[0]('index'))) # printing the result
测试结果的平均误差为 2.66e-07。
希望我的经验对你们有用,祝你们好运。
关注公众号:拾黑(shiheibook)了解更多
[广告]赞助链接:
四季很好,只要有你,文娱排行榜:https://www.yaopaiming.com/
让资讯触达的更精准有趣:https://www.0xu.cn/
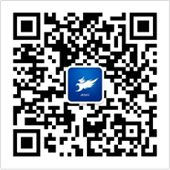
随时掌握互联网精彩
- 1 习近平G20里约峰会展现大国担当 7943276
- 2 多国驻乌克兰大使馆因袭击风险关闭 7931897
- 3 78岁老太将减持2.5亿股股票 7885988
- 4 二十国集团里约峰会将会卓有成效 7779115
- 5 俄导弹击中乌水电站大坝 7666759
- 6 孙颖莎王艺迪不敌日本削球组合 7584961
- 7 高三女生酒后被强奸致死?检方回应 7449882
- 8 第一视角记录虎鲨吞下手机全程 7305910
- 9 73岁王石独自带娃被偶遇 7271961
- 10 智慧乌镇点亮数字经济新未来 7125597