作者:Abhishek Sharma 翻译:李嘉骐 校对:方星轩本文长度为5500字,建议阅读10+分钟
本文利用Python对Amazon产品的反馈对数据文本进行探索性研究与分析,并给出结论。
在一个机器学习项目的全流程中是没有捷径可走的,比如我们无法在收集齐所需的数据后直接跳到模型搭建的阶段。我们需要有条理地规划方法,而在此过程中探索性数据分析(EDA)阶段是十分重要的。在我从事这一领域的前期,总是急切地要深入研究机器学习算法,但这常常得到不确定性的结果。通过个人经历和导师的建议,我意识到在探索和理解数据上花时间是必要的。
即使是自然语言处理(NLP)项目[1]中的文本数据也是如此。我们需要对数据进行研究和探索性分析,看看是否能挖掘出有意义的发现。相信我,处理的文本数据越多,你就会感激EDA这个过程。当然我们也不缺文本数据。我们有来自推特、数字媒体平台、博客和其他大量来源的数据。作为一个数据科学家和NLP爱好者,分析这些文本数据以帮助你的公司做出数据驱动的决策非常重要。探索性的数据分析将这些紧密联系在一起。可以说这是NLP项目中的一个关键环节——一个你根本无法跳过的阶段。-探索性数据分析是探索数据、形成见解、检验假设、检查预设条件并揭示数据中潜在的隐藏规律的过程。-(Exploratory Data Analysis is the process of exploring data, generating insights, testing hypotheses, checking assumptions and revealing underlying hidden patterns in the data.)因此,在本文中,我们将通过一个实际的例子讨论如何使用Python对文本数据进行探索性数据分析。如果你不熟悉NLP或数据可视化的辉煌而广阔的世界,可以浏览以下资源:https://courses.analyticsvidhya.com/courses/tableau-2-0https://courses.analyticsvidhya.com/courses/Intro-to-NLP对于任何一个机器学习项目,第一步就是理解问题。这也是我们本章要讨论的内容。我是亚马逊系列产品的超级粉丝。我这里有一个数据集,其中包含了亚马逊的各种产品的评论,如Kindle、Fire TV、Echo等。https://www.kaggle.com/datafiniti/consumer-reviews-of-amazon-products
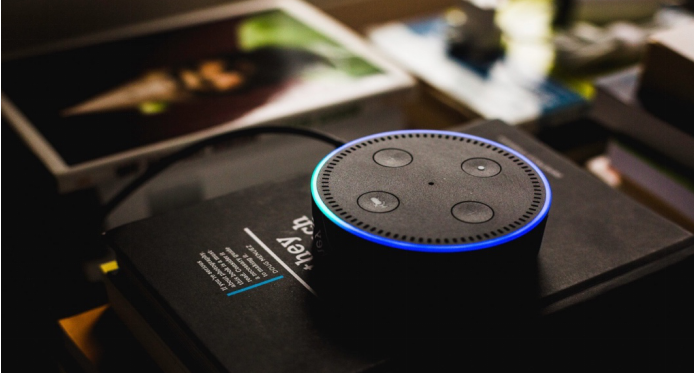
该数据集大有34000多行,每行包含每个产品的评论文本、用户名、产品名称、评级和其他信息。我们的目标是使用这些数据,探索它,并从中形成自己的见解。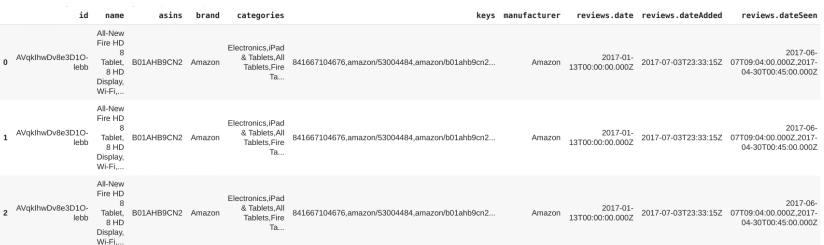
让我们开始使用不同的技术来研究这个数据集,并从中形成自己的见解。在进入数据探索阶段之前,我们需要进行基本的数据预处理,如空值插补和去除不需要的数据。那么,让我们从导入库和读取数据集开始:import numpy as np
import pandas as pd
# 可视化
import matplotlib.pyplot as plt
# 正则化
import re
# 处理字符串
import string
# 执行数学运算
import math
# 导入数据
df=pd.read_csv('dataset.csv') print("Shape of data=>",df.shape)

数据集包含34660行和21列。但我们只需要诸如产品名称、评论文本、用户推荐(推荐或不推荐)和认为该评论有用的人数等信息。因此,我删除了其他列,并将数据集缩减为只有四列,即“名称”、“评论文本”, “评论-是否推荐”和“评论-认为此评论有用的人数”:df=df[['name','reviews.text','reviews.doRecommend','reviews.numHelpful']]
print("Shape of data=>",df.shape)
df.head(5)
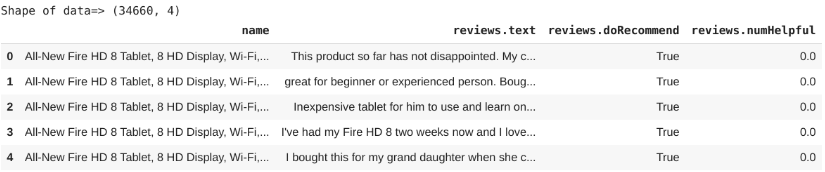

数据集中存在一些空值,所以我们删除掉这些空值后再往下进行:df.dropna(inplace=True)
df.isnull().sum()

我只利用至少有500条评论的产品。这样做是为了确保每个产品都有足够数量的评论。这里,我们可以使用lambda函数和filter()来过滤数据集。如果你想了解更多关于lambda函数,可以阅读这篇文章:什么是lambda函数?Python中Lambda函数的快速指南。我强烈建议去阅读这篇指南,因为本文之后部分将会多次使用lambda函数。什么是lambda函数?Python中Lambda函数的快速指南:https://www.analyticsvidhya.com/blog/2020/03/what-are-lambda-functions-in-python/df=df.groupby('name').filter(lambda x:len(x)>500).reset_index(drop=True)
print('Number of products=>',len(df['name'].unique()))

现在只剩下了八种产品。另外,“评论-是否推荐”列包含二值化数据True-False; '“评论-认为此评论有用的人数”列包含浮点数,这些数据类型不能直接用来处理。因此,我将这些列的数据类型转换为整数:df['reviews.doRecommend']=df['reviews.doRecommend'].astype(int)
df['reviews.numHelpful']=df['reviews.numHelpful'].astype(int)
就这样!基本的数据预处理部分已经完成。让我们转到下一节—清洗文本数据。一般来说,文本数据包含大量干扰项以符号或者标点符号及停用词的形式出现。因此对文本进行清洗是必要的,不仅为了使其更易于理解,而且为了从中获得更好的发现。在本节中,我们将对包含文本数据的列执行文本清洗。如果你想深入了解Python中的文本清洗,可以阅读以下这篇很棒的文章,它解释了各种文本清洗技术,并给出了基于Python的代码实现。有效文本数据清洗的步骤(通过使用Python的案例研究:https://www.analyticsvidhya.com/blog/2014/11/text-data-cleaning-steps-python/我们的数据集中有四列,其中两列(“名称”,“评论文本”包含文本数据。所以,让我们先从“名称”列开始,看看这一列中的文本:
仔细看看产品名称。某些产品名称包含由三个连续逗号(,,,)分隔的重复名称。因此,我们对产品名称做一下文本清洗:df['name']=df['name'].apply(lambda x: x.split(',,,')[0])
现在可以去处理第二栏了——“评论文本”. 本栏包含不同用户的产品评论,篇幅较长,我们的完整分析也将基于评论文本。因此,有必要对其进行彻底清洗。确定数据清洗步骤的最佳方法是从数据集中查看一些产品评论: for index,text in enumerate(df['reviews.text'][35:40]):
print('Review %d:\n'%(index+1),text)
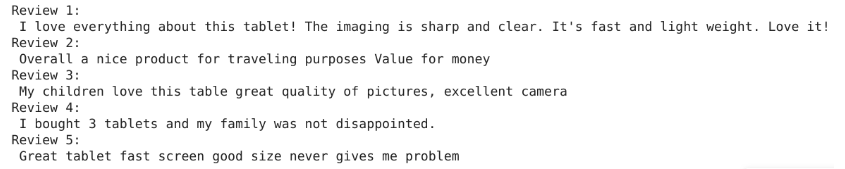
可以看到有一些在评论中出现的缩略语,比如“It's”;数字,比如“3”;标点符号,比如“,”,“!”,“.”。我们将通过下面的操作来处理这些问题:扩展缩略语;
将评论文本小写;
删除数字和包含数字的单词;
删除标点符号。
缩略语是单词的缩写,比如don't代表do not,how'll代表how will。人们使用缩略语来减少单词的读写时间。我们需要扩展这些缩略语,以便更好地分析评论。这里创建了一个常用英语缩略语词典,将用于把缩略语映射到它们的扩展形式: # Dictionary of English Contractions
contractions_dict = { "ain't": "are not","'s":" is","aren't": "are not",
"can't": "cannot","can't've": "cannot have",
"'cause": "because","could've": "could have","couldn't": "could not",
"couldn't've": "could not have", "didn't": "did not","doesn't": "does not",
"don't": "do not","hadn't": "had not","hadn't've": "had not have",
"hasn't": "has not","haven't": "have not","he'd": "he would",
"he'd've": "he would have","he'll": "he will", "he'll've": "he will have",
"how'd": "how did","how'd'y": "how do you","how'll": "how will",
"I'd": "I would", "I'd've": "I would have","I'll": "I will",
"I'll've": "I will have","I'm": "I am","I've": "I have", "isn't": "is not",
"it'd": "it would","it'd've": "it would have","it'll": "it will",
"it'll've": "it will have", "let's": "let us","ma'am": "madam",
"mayn't": "may not","might've": "might have","mightn't": "might not",
"mightn't've": "might not have","must've": "must have","mustn't": "must not",
"mustn't've": "must not have", "needn't": "need not",
"needn't've": "need not have","o'clock": "of the clock","oughtn't": "ought not",
"oughtn't've": "ought not have","shan't": "shall not","sha'n't": "shall not",
"shan't've": "shall not have","she'd": "she would","she'd've": "she would have",
"she'll": "she will", "she'll've": "she will have","should've": "should have",
"shouldn't": "should not", "shouldn't've": "should not have","so've": "so have",
"that'd": "that would","that'd've": "that would have", "there'd": "there would",
"there'd've": "there would have", "they'd": "they would",
"they'd've": "they would have","they'll": "they will",
"they'll've": "they will have", "they're": "they are","they've": "they have",
"to've": "to have","wasn't": "was not","we'd": "we would",
"we'd've": "we would have","we'll": "we will","we'll've": "we will have",
"we're": "we are","we've": "we have", "weren't": "were not","what'll": "what will",
"what'll've": "what will have","what're": "what are", "what've": "what have",
"when've": "when have","where'd": "where did", "where've": "where have",
"who'll": "who will","who'll've": "who will have","who've": "who have",
"why've": "why have","will've": "will have","won't": "will not",
"won't've": "will not have", "would've": "would have","wouldn't": "would not",
"wouldn't've": "would not have","y'all": "you all", "y'all'd": "you all would",
"y'all'd've": "you all would have","y'all're": "you all are",
"y'all've": "you all have", "you'd": "you would","you'd've": "you would have",
"you'll": "you will","you'll've": "you will have", "you're": "you are",
"you've": "you have"}
# Regular expression for finding contractions
contractions_re=re.compile('(%s)' % '|'.join(contractions_dict.keys()))
# Function for expanding contractions
def expand_contractions(text,contractions_dict=contractions_dict):
def replace(match):
return contractions_dict[match.group(0)]
return contractions_re.sub(replace, text)
# Expanding Contractions in the reviews
df['reviews.text']=df['reviews.text'].apply(lambda x:expand_contractions(x))
在这里,expand_constrations函数使用正则表达式将文本中的缩率词映射到字典中它们的扩展形式。在接下来的章节中将大量使用正则表达式。因此,强烈建议你阅读以下有关正则表达式的文章:https://www.analyticsvidhya.com/blog/2015/06/regular-expression-python/https://www.analyticsvidhya.com/blog/2017/03/extracting-information-from-reports-using-regular-expressons-library-in-python/每个数据科学家都应知道的4个正则表达式应用(用Python代码实现)https://www.analyticsvidhya.com/blog/2020/01/4-applications-of-regular-expressions-that-every-data-scientist-should-know-with-python-code/扩展缩略词部分已经完成,现在我们去尝试把单词变成小写的形式。在NLP中,即使Goat和goat两个单词是相同的,模型也是将它们作为不同单词来处理的。因此,为了克服这个问题,我们将单词变为小写形式。作者使用Python中的lower()函数将文本转换为小写:df['cleaned']=df['reviews.text'].apply(lambda x: x.lower())
接下来,我们需要从评论中删除数字和包含数字的单词,因为数字和包含数字的单词对主要整句话来说意义不大。为此,作者借助lambda函数使用了正则表达式。df['cleaned']=df['cleaned'].apply(lambda x: re.sub('\w*\d\w*','', x))
标点符号是英语中的标记,如逗号、连字符、句号等。它们对英语语法很重要,但对文本分析却不重要。因此需要将其移除:df['cleaned']=df['cleaned'].apply(lambda x: re.sub('[%s]' % re.escape(string.punctuation), '', x))
在这里,string.punctuations函数包含所有标点符号,我们使用正则表达式在文本中搜索并删除它们。最后,我们在数据中还有一些额外的空格,我们也一并删除它们: # Removing extra spaces
df['cleaned']=df['cleaned'].apply(lambda x: re.sub(' +',' ',x))
for index,text in enumerate(df['cleaned'][35:40]):
print('Review %d:\n'%(index+1),text)
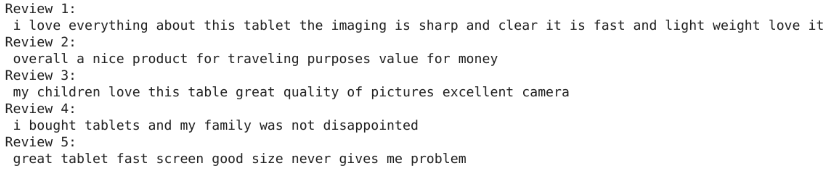
太好了!我们已经完成了文本数据的清洗,离EDA阶段只差一节了!我们已经清洗了数据并准备好了语料库,但是在EDA之前还有一些步骤要做。在本节中,我们将创建一个文档术语矩阵,并在稍后的分析中加以使用。现在你可能想知道什么是文档术语矩阵(Document Term Matrix),以及为什么我们需要创建它。文档术语矩阵提供了一个词在语料库(文档集合)中的频率,在本例中指的是评论。它有助于分析语料库中不同文档中单词的出现情况。下图是文档术语矩阵的示例:
停用词(stopwords) 是像“I”、“this”、“is”、“in”这样的最常见的单词,它们在文档中的含义不大。删除这些值可以减小数据集的大小并增加对有意义单词的关注度。词形还原(Lemmatization)是一个系统的过程,它将单词还原成其基本的形式。它使用词汇、词结构、词性标记和语法关系将单词转换为其基本形式。你可以阅读这篇文章获得更多关于删除停用词和词形还原的内容:NLP要点:在Python中使用NLTK和spaCy来删除停用词与规范化文本:https://www.analyticsvidhya.com/blog/2019/08/how-to-remove-stopwords-text-normalization-nltk-spacy-gensim-python/我们将使用SpaCy来完成停用词删除和词形还原。它是一个用Python和Cython进行高级自然语言处理的库。 # Importing spacy
import spacy
# Loading model
nlp = spacy.load('en_core_web_sm',disable=['parser', 'ner'])
# Lemmatization with stopwords removal
df['lemmatized']=df['cleaned'].apply(lambda x: ' '.join([token.lemma_ for token in list(nlp(x)) if (token.is_stop==False)]))
我们已经成功地删除了停用词并对我们的评论做了词形还原。我们根据产品对这些评论分组:df_grouped=df[['name','lemmatized']].groupby(by='name').agg(lambda x:' '.join(x))
df_grouped.head()
现在可以创建文档术语矩阵了。你可以在以下阅读更多关于文档术语矩阵的信息:https://www.analyticsvidhya.com/blog/2020/02/quick-introduction-bag-of-words-bow-tf-idf # Creating Document Term Matrix
from sklearn.feature_extraction.text
import CountVectorizer cv=CountVectorizer(analyzer='word')
data=cv.fit_transform(df_grouped['lemmatized'])
df_dtm = pd.DataFrame(data.toarray(), columns=cv.get_feature_names())
df_dtm.index=df_grouped.index
df_dtm.head(3)
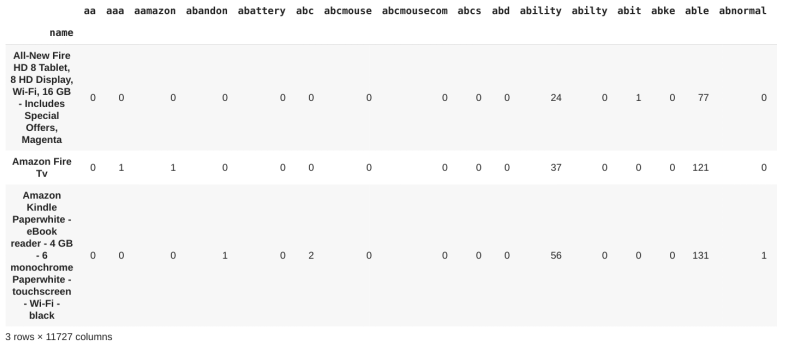
最后,在开始分析文本之前,我们已经完成了所需的所有预处理步骤,并且使数据集以探索阶段所需的格式呈现。基于Python的Amazon产品评论探索性数据分析是的——终于到了探索性数据分析的时候了!这是任何数据科学项目的重要组成部分,因为在这一步中你会更多地了解数据。在这个阶段,你可以发掘数据中隐藏的规律,并从中形成自己的见解。让我们从每个产品的评论中常见的词语开始。这里将使用前面创建的文档术语矩阵,以词云(Word Clouds)来可视化这些单词。词云是文档中不同单词出现频率的直观表示。它将更频繁出现的单词以更大的尺寸显示出来。词云可以使用wordcloud库生成。我们为每个产品绘制词云:# Importing wordcloud for plotting word clouds and textwrap for wrapping longer text
from wordcloud import WordCloud
from textwrap import wrap
# Function for generating word clouds
def generate_wordcloud(data,title):
wc = WordCloud(width=400, height=330, max_words=150,colormap="Dark2").generate_from_frequencies(data)
plt.figure(figsize=(10,8))
plt.imshow(wc, interpolation='bilinear')
plt.axis("off")
plt.title('\n'.join(wrap(title,60)),fontsize=13)
plt.show()
# Transposing document term matrix
df_dtm=df_dtm.transpose()
# Plotting word cloud for each product
for index,product in enumerate(df_dtm.columns):
generate_wordcloud(df_dtm[product].sort_values(ascending=False),product)

可以看到,几乎每一个产品,LOVE,USE,BUY,GREAT和EASY是最频繁出现的词。这意味着用户喜欢亚马逊的产品,并发现购买它们是一个很棒的决定。他们还发现它们易于使用。假设亚马逊想更深入地研究这个问题,并想知道他们应该改进哪些产品。我们可以通过对每个产品的评论进行情感分析来找到这个问题的答案。情感分析分析一段文本是正面或是负面。如果你想了解更多关于情感分析的知识可以参考这篇文章:全面的Twitter情绪分析实践指南,包含数据集和代码:https://www.analyticsvidhya.com/blog/2018/07/hands-on-sentiment-analysis-dataset-python/对于我们的问题,只要检查评论的极性就足够了,即文本给出多么正面或负面的评价。我们可以使用Python中的TextBlob库检查评论的极性: from textblob import TextBlob
df['polarity']=df['lemmatized'].apply(lambda x:TextBlob(x).sentiment.polarity)
print("3 Random Reviews with Highest Polarity:")
for index,review in enumerate(df.iloc[df['polarity'].sort_values(ascending=False)[:3].index]['reviews.text']):
print('Review {}:\n'.format(index+1),review)

print("3 Random Reviews with Lowest Polarity:")
for index,review in enumerate(df.iloc[df['polarity'].sort_values(ascending=True)[:3].index]['reviews.text']):
print('Review {}:\n'.format(index+1),review)

我们来画出每个产品的评论的极性并进行比较。条形图最适合于此目的: product_polarity_sorted=pd.DataFrame(df.groupby('name')['polarity'].mean().sort_values(ascending=True))
plt.figure(figsize=(16,8))
plt.xlabel('Polarity')
plt.ylabel('Products')
plt.title('Polarity of Different Amazon Product Reviews')
polarity_graph=plt.barh(np.arange(len(product_polarity_sorted.index)),product_polarity_sorted['polarity'],color='purple',)
# Writing product names on bar
for bar,product in zip(polarity_graph,product_polarity_sorted.index):
plt.text(0.005,bar.get_y()+bar.get_width(),'{}'.format(product),va='center',fontsize=11,color='white')
# Writing polarity values on graph
for bar,polarity in zip(polarity_graph,product_polarity_sorted['polarity']):
plt.text(bar.get_width()+0.001,bar.get_y()+bar.get_width(),'%.3f'%polarity,va='center',fontsize=11,color='black')
plt.yticks([])
plt.show()

可以看到,根据评论的极性,亚马逊需要改进Fire Kids Edition Tablet和Kindle Voyage E-reader两款产品。我们也来看看推荐某个产品的用户数量。为此可以获取评价者的百分比并可视化: recommend_percentage=pd.DataFrame(((df.groupby('name')['reviews.doRecommend'].sum()*100)/df.groupby('name')['reviews.doRecommend'].count()).sort_values(ascending=True))
plt.figure(figsize=(16,8))
plt.xlabel('Recommend Percentage')
plt.ylabel('Products')
plt.title('Percentage of reviewers recommended a product')
recommend_graph=plt.barh(np.arange(len(recommend_percentage.index)),recommend_percentage['reviews.doRecommend'],color='green')
# Writing product names on bar
for bar,product in zip(recommend_graph,recommend_percentage.index):
plt.text(0.5,bar.get_y()+0.4,'{}'.format(product),va='center',fontsize=11,color='white')
# Writing recommendation percentage on graph
for bar,percentage in zip(recommend_graph,recommend_percentage['reviews.doRecommend']):
plt.text(bar.get_width()+0.5,bar.get_y()+0.4,'%.2f'%percentage,va='center',fontsize=11,color='black')
plt.yticks([])
plt.show()

可以看到 Fire Kids Edition Tablet 的推荐比例最低。它的评论也是最负面的。因此评论的极性影响了产品获得推荐的可能性。我们也可以去看看评论的可读性,即评论被其他用户认为是有帮助的。我们可以使用各种可读性指标检查文本文档的可读性,比如Flesch阅读容易度、Dale Chall可读性评分和Gunning Fog指数。为此,我们可以使用Python中的textstat库。Textstat通常用于判断特定语料库的可读性、复杂性和等级。其中的每一个指标都使用不同的方法来确定文档的可读性级别。 recommend_percentage=pd.DataFrame(((df.groupby('name')['reviews.doRecommend'].sum()*100)/df.groupby('name')['reviews.doRecommend'].count()).sort_values(ascending=True))
import textstat
df['dale_chall_score']=df['reviews.text'].apply(lambda x: textstat.dale_chall_readability_score(x))
df['flesh_reading_ease']=df['reviews.text'].apply(lambda x: textstat.flesch_reading_ease(x))
df['gunning_fog']=df['reviews.text'].apply(lambda x: textstat.gunning_fog(x))
print('Dale Chall Score of upvoted reviews=>',df[df['reviews.numHelpful']>1]['dale_chall_score'].mean())
print('Dale Chall Score of not upvoted reviews=>',df[df['reviews.numHelpful']<=1]['dale_chall_score'].mean())
print('Flesch Reading Score of upvoted reviews=>',df[df['reviews.numHelpful']>1]['flesh_reading_ease'].mean())
print('Flesch Reading Score of not upvoted reviews=>',df[df['reviews.numHelpful']<=1]['flesh_reading_ease'].mean())
print('Gunning Fog Index of upvoted reviews=>',df[df['reviews.numHelpful']>1]['gunning_fog'].mean())
print('Gunning Fog Index of not upvoted reviews=>',df[df['reviews.numHelpful']<=1]['gunning_fog'].mean())
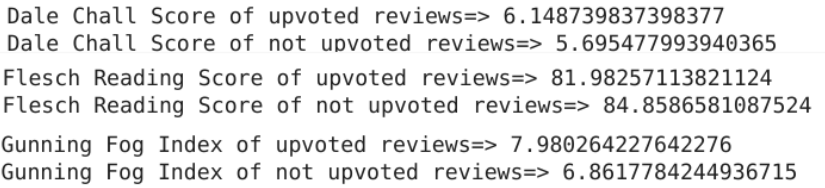
在有帮助的和没有帮助的评论中, Dale Chall评分和Flesch阅读评分几乎没有差别。但Gunning Fog指数差别相当大。截至目前,我们还是无法区分两者的可读性。textstat库也有一个解决方案。它提供text_standard()函数。该函数使用各种可读性检查公式,综合所有结果并返回完全理解特定文档所需的受教育程度。 df['text_standard']=df['reviews.text'].apply(lambda x: textstat.text_standard(x))
print('Text Standard of upvoted reviews=>',df[df['reviews.numHelpful']>1]['text_standard'].mode())
print('Text Standard of not upvoted reviews=>',df[df['reviews.numHelpful']<=1]['text_standard'].mode())

结果很有趣,被认为有用和没有用的评论都很容易被五六年级学生水平的人理解。我们来看看有帮助和没有帮助的评论的所需阅读时间。一个成年人平均每分钟阅读250个单词。我们还可以使用textstat库计算文档的读取时间。它提供reading_time()函数,该函数将一段文本作为参数,并以秒为单位返回该文本的读取时间。 df['reading_time']=df['reviews.text'].apply(lambda x: textstat.reading_time(x))
print('Reading Time of upvoted reviews=>',df[df['reviews.numHelpful']>1]['reading_time'].mean())
print('Reading Time of not upvoted reviews=>',df[df['reviews.numHelpful']<=1]['reading_time'].mean())

令人惊讶的是,被认为有用的评论的阅读时间是被认为没有用的评论的两倍。这意味着人们通常认为较长的评论更有帮助。顾客喜欢亚马逊的产品。它们令人满意同时易于使用;
亚马逊需要提升 Fire Kids Edition Tablet 这款产品,因为它的负面评论最多。它也是最不被推荐的产品;
大部分的评论都是用简单的英语写的,任何一个五六年级水平的人都很容易理解;
有用的评论的阅读时间是非有用评论的两倍,这意味着人们发现较长的评论更有帮助。
需要注意的的是探索性数据分析没有固定的方法。它完全取决于你拥有的数据、需要解决的问题的描述以及领域知识。一组完美地处理一个数据的流程可能无法处理另一个数据。掌握探索性数据分析的唯一方法是分析来自不同领域的不同数据集。如果你觉得这篇文章内容丰富,请向你的朋友们分享,也欢迎在留言区给出你的问题与反馈。下面列出一些与自然语言处理相关的有见地和综合性的文章和课程。https://www.analyticsvidhya.com/blog/category/nlp/https://courses.analyticsvidhya.com/courses/Intro-to-NLPNLhttps://www.analyticsvidhya.com/blog/2020/01/learning-path-nlp-2020/利用词袋模型(BoW)和TF-IDF从文本创建特征的快速介绍https://www.analyticsvidhya.com/blog/2020/02/quick-introduction-bag-of-words-bow-tf-idf/https://www.analyticsvidhya.com/blog/2020/03/pretrained-word-embeddings-nlp/你应该知道的重要自然语言处理框架的完整列表(NLP Infographic)https://www.analyticsvidhya.com/blog/2019/08/complete-list-important-frameworks-nlp/A Beginner’s Guide to Exploratory Data Analysis (EDA) on Text Data (Amazon Case Study)https://www.analyticsvidhya.com/blog/2020/04/beginners-guide-exploratory-data-analysis-text-data/译者简介:李嘉骐,清华大学自动化系博士在读,本科毕业于北京航空航天大学自动化学院,研究方向为生物信息学。对数据分析和数据可视化有浓厚兴趣,喜欢运动和音乐。
「完」
版权声明:本号内容部分来自互联网,转载请注明原文链接和作者,如有侵权或出处有误请和我们联系。
数据分析(ID : ecshujufenxi )互联网科技与数据圈自己的微信,也是WeMedia自媒体联盟成员之一,WeMedia联盟覆盖5000万人群。关注公众号:拾黑(shiheibook)了解更多
[广告]赞助链接:
四季很好,只要有你,文娱排行榜:https://www.yaopaiming.com/
让资讯触达的更精准有趣:https://www.0xu.cn/